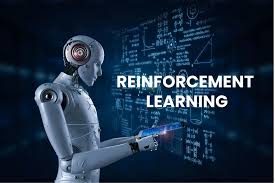
While RL has already made significant strides in various sectors, its full potential is still largely untapped. In the coming years, we can expect several key trends to shape the future of reinforcement learning.
1. Improved Sample Efficiency and Generalization
One of the biggest challenges in RL is the sample inefficiency—RL algorithms often require vast amounts of data and interactions with the environment to learn effectively. Training an RL agent can take a long time and require enormous computational resources. For example, training AI models to play games like Dota 2 or Go involves millions of simulations.
Researchers are actively working on improving sample efficiency, which would allow RL models to learn faster with fewer interactions. Advances in techniques like meta-learning (learning to learn) and transfer learning (applying knowledge gained in one context to another) are already showing promise in this regard. These innovations could significantly reduce the time and resources needed to train RL systems, enabling them to be deployed in real-world applications more efficiently.
2. Integration with Other AI Techniques
In the future, RL is likely to be integrated with other AI techniques, such as supervised learning, unsupervised learning, and neural networks, to create hybrid models that leverage the strengths of each approach. For example, combining RL with deep learning (deep reinforcement learning) has already led to breakthroughs in complex games and robotics.
Integrating RL with other approaches could enhance the versatility of AI systems, enabling them to handle a wider range of tasks, from perception and natural language understanding to reasoning and decision-making.
3. Real-World Applications and Deployment
As RL becomes more efficient and scalable, we can expect it to be deployed in more complex and high-stakes real-world applications. For example, RL could be used in sectors like healthcare to optimize patient care in real-time, in energy management to balance supply and demand dynamically, or in autonomous manufacturing to manage large-scale production systems.
Moreover, RL could play a significant role in smart cities, where it could help optimize traffic flow, manage public utilities, and improve urban planning. As cities become smarter and more connected, RL could help create more efficient, sustainable, and livable urban environments.
4. Ethical Considerations and Safety
As RL-based systems are deployed in critical sectors, safety and ethics will become increasingly important. RL systems learn by exploring different actions, but there’s a risk that they might explore harmful or unsafe actions if not properly constrained. This is known as the exploration-exploitation tradeoff.
Researchers are working on creating safe reinforcement learning algorithms that ensure AI agents behave safely in unpredictable or complex environments. Furthermore, ethical considerations such as fairness, transparency, and accountability will need to be addressed as RL systems make decisions that affect human lives.
5. AI-Driven Creativity and Design
In the future, RL could play a significant role in the creative industries, helping to design new products, create art, or even compose music. By exploring different creative approaches and receiving feedback, RL agents could work alongside human creators to generate innovative and novel ideas.
For example, RL could be used to design complex architectural structures, optimize visual arts for specific aesthetics, or assist in the creation of personalized content in gaming or film production.
Conclusion: A Bright Future for Reinforcement Learning
Reinforcement learning has come a long way, and its potential to revolutionize various industries is only beginning to be realized. As RL algorithms become more efficient, scalable, and integrated with other AI techniques, we can expect them to play an increasingly important role in areas like robotics, healthcare, finance, manufacturing, and even creative fields.
The future of reinforcement learning is exciting, but it also comes with challenges. To unlock its full potential, we must ensure that RL systems are safe, ethical, and capable of learning in complex, real-world environments. As we continue to push the boundaries of AI, reinforcement learning will undoubtedly be at the forefront of innovation, driving the next wave of intelligent, autonomous systems.
Whether you’re a developer, researcher, or simply an AI enthusiast, keeping an eye on the evolution of reinforcement learning will be crucial as it shapes the future of artificial intelligence.